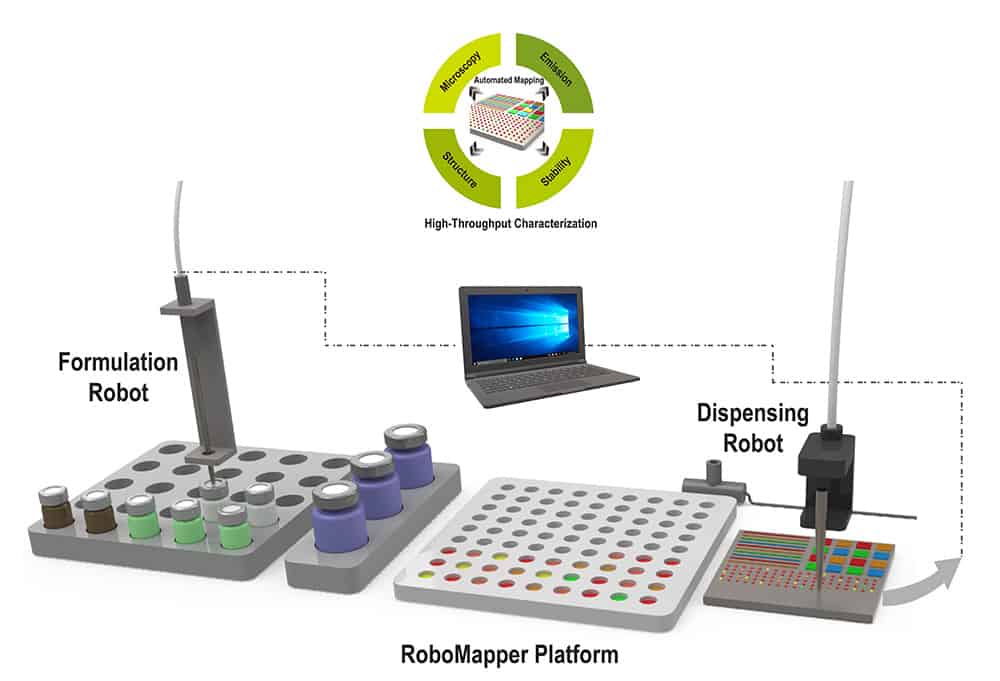
[Image above] Schematic of the RoboMapper platform that enabled automated ink formulation and microprinting of a wide variety of solution-processable materials from a library of precursors onto a common substrate. The materials array is then subjected to multimodal characterization and mapping using a wide range of laboratory- and synchrotron-based microprobes. Photo courtesy Aram Amassian. Credit: Wang et al., Matter
The potential of perovskite-based solar technologies is gaining steam, as demonstrated on CTT last Friday. Within just the past year, multiple records were set and surpassed for both perovskite–silicon tandem and all-perovskite solar cells.
Computer simulations are one reason perovskite developments are occurring at such a rapid pace. They allow promising compositions and structures to be determined more quickly than testing every option through conventional trial-and-error experiments.
Once promising perovskites are identified, however, conventional trial-and-error experiments are needed on the different compositions to verify the predictions. These tests can be repetitive and labor intensive, and thus limit the speed of the development process.
In recent years, researchers have started using advanced computing techniques and robotic systems to automate the experimental steps of the development process. To date, these efforts have relied largely on automating the existing assembly line structure, i.e., samples are sent one by one through the entire data collection process.
Advances in capillary printing and liquid handling, such as here and here, means researchers can now design systems that robotically deposit multiple samples on a single substrate. This format allows data collection to take place on multiple materials in parallel, thus saving time and energy.
In a recent paper, researchers led by North Carolina State University described their new multisample, high-throughput automated testing system called the RoboMapper. The compact, benchtop system consists of an ink formulation bot that works in tandem with a dispensing bot, which allows microprinting and microcoating with volumes ranging from 0.6 pL to 0.5 nL.
In contrast to other automated systems, the RoboMapper platform deposits samples using a gantry-based hollow capillary approach rather than spin-coating or drop-casting. This approach allows for the creation of smaller sample areas, which leads to lower costs.
To test the utility of the RoboMapper platform, the researchers used it to search for perovskite compositions that can best withstand degradation when exposed to sunlight.
With each composition taking about 8 minutes to make, they ultimately deposited 150 different metal halide perovskite samples on one substrate using the RoboMapper system. They then evaluated these samples using optical spectroscopy and X-ray structural assessments. Results from the RoboMapper process were validated using conventional testing techniques.
In the paper, the researchers describe the significant time, cost, and energy savings that came with using the RoboMapper platform instead of serial (one-by-one) automation or a manual approach, as well as the reduced environmental impact.
- Time savings. Thanks to the reduced number of operational steps associated with sample loading, alignment, and calibration, it can take as little as 6 days for 500 compositions to be palletized and fully screened through the RoboMapper platform. In contrast, the same task requires at least 54 days via serial automation (9-fold increase) and upward of 84 days for a manual workflow based on full-time labor (14-fold increase).
- Cost and energy savings. The researchers estimate that a sample dataset generated through the RoboMapper workflow will only cost $0.34 and 0.344 kWh, in contrast to $2.82 and 6.284 kWh for existing serial automation workflows and $17.61 and 8.936 kWh for manual workflows.
- Environmental impact. Due to the streamlined data collection that comes from the RoboMapper system placing dozens of samples on the same substrate, the researchers determined this approach has a significantly lower environmental impact than serial automation or a manual approach. They provide estimates for reduced waste generation and greenhouse gas emissions as examples.
In a press release, Aram Amassian, professor of materials science and engineering at North Carolina State University, says the group plans to use the RoboMapper platform to expedite research on other solution-processable materials, such as organic semiconductors, quantum dots, and nanoparticles.
“We’re open to working with industry partners to identify new materials for photovoltaics or other applications. And with support from the Office of Naval Research, we are already using RoboMapper to advance our understanding of materials for both organic solar cells and printed electronics,” he says.
This work contributes to the goal of eventually creating a fully autonomous materials acceleration platform (MAP). MAPs are envisioned as close-looped systems that use computer models to determine the parameters of an experiment, which are then conducted on an automated robotic platform. Data from the experiment is then fed back into the model, which will propose new experiments for execution on the robotic platform. This process would continue indefinitely until desired results are achieved.
The paper, published in Matter, is “Sustainable materials acceleration platform reveals stable and efficient wide-bandgap metal halide perovskite alloys” (DOI: 10.1016/j.matt.2023.06.040).